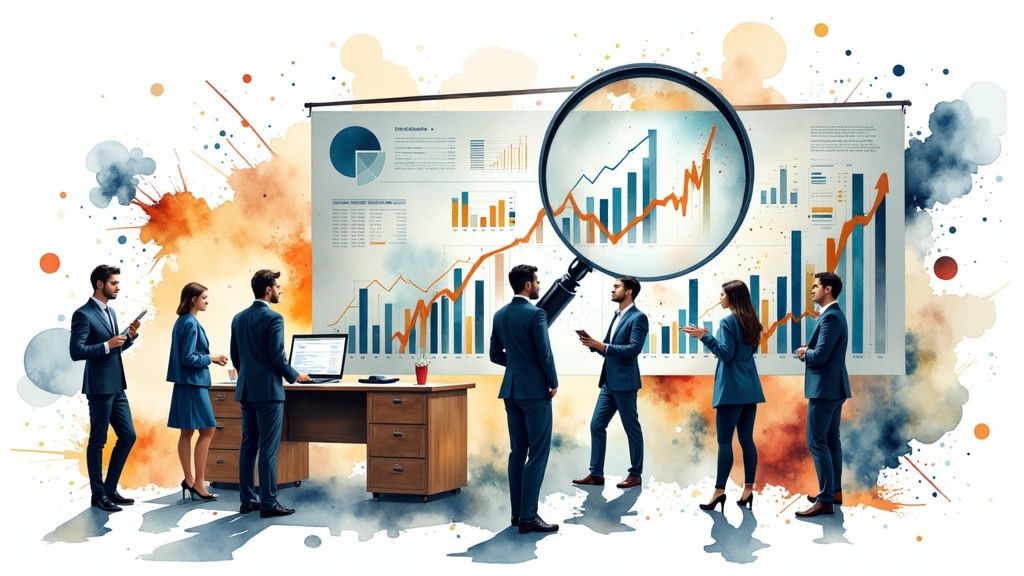
5 Powerful Data Driven Decision Making Examples From Leading Organizations
The Art and Science of Data Driven Decision Making
Organizations are increasingly moving away from gut instinct and toward evidence-based leadership. This shift combines human insight with data analysis to create better business outcomes. By examining real examples from successful companies, we can see how they've built lasting data practices that deliver results.
Insights from Leading Organizations
Recent data shows the growing adoption of data-driven approaches across industries. A 2024 survey revealed that 63% of marketing teams found moderate success with their data strategies, while 32% reported high success. Only 5% saw poor results. The benefits are clear – 25% of companies now use data for most strategic choices, leading to 8% higher revenues and 10% lower costs on average. Find more detailed statistics here.
Overcoming Challenges
Two main hurdles often stand in the way of data-driven success:
-
Resistance to Change: Some staff members prefer traditional decision-making methods. The key is creating a culture where decisions come from facts rather than opinions.
-
Choosing the Right Metrics: Not all data points matter equally. Success depends on picking Key Performance Indicators (KPIs) that match company goals.
This table shows how metric selection affects outcomes:
Metric Approach | Outcome |
---|---|
Right Metrics | Informed decisions, growth |
Wrong Metrics | Misguided strategies, stagnation |
Practical Frameworks for Success
Follow these steps to build data into your organization:
-
Define Objectives: Begin with clear goals that will guide what data you collect and analyze.
-
Cultivate a Data Culture: Build trust in data through training and education programs.
-
Implement and Iterate: Put data strategies into action and keep improving based on results.
Companies that follow these principles see better outcomes in their decision-making. Tools like Derisky.ai can help organizations gain an edge by putting these frameworks into practice effectively.
Inside Walmart's Data Revolution: Transforming Retail Through Analytics
As the world's largest retailer, Walmart has fundamentally changed how it operates by putting data at the center of its business. The company's shift from traditional retail practices to data-informed decision making shows how analytics can improve every aspect of operations, from stocking shelves to predicting customer demand.
Inventory Optimization and Demand Forecasting
Smart inventory management drives Walmart's success. By studying past sales patterns and using predictive models, store managers can better stock what customers want, when they want it. During busy holiday seasons, this means getting the right mix of seasonal items to each location. The result? Fewer empty shelves, less excess inventory, and happier shoppers who find what they need. Learn more about data-driven retail.
Practical Approaches to Data Collection
Walmart gathers insights through several key methods:
-
Customer Data: Tracking purchases, shopping patterns, and social media conversations
-
Performance Metrics: Following specific measures that connect to business goals, like how quickly products sell and how often items need restocking
-
Action Plans: Converting data findings into concrete changes for product selection, store layouts, and marketing
Tools and Technologies Employed
Walmart relies on several advanced systems working together:
- Machine Learning and AI: To analyze data and make predictions in real-time
- Cloud Computing: For storing and accessing data across locations
- Internet of Things (IoT): To track products and monitor store conditions
While Walmart has seen great results, they've also faced real challenges around protecting customer privacy and managing their vast data systems. Their experience shows that success requires constant learning and adapting to new data opportunities.
By focusing on practical uses of data, Walmart demonstrates how thoughtful analysis can improve business results and create better shopping experiences for customers.
How Salesforce Revolutionized Product Development Through Customer Data
Salesforce shows how companies can excel by putting customer data at the heart of product development. Their success comes from directly involving customers in shaping products, creating strong partnerships that benefit everyone involved. This approach has helped them stay ahead in the competitive CRM industry by consistently delivering exactly what customers need.
The IdeaExchange: A Platform for Customer Collaboration
The IdeaExchange platform, launched in 2006, is central to how Salesforce gathers and uses customer input. Rather than just being a simple suggestion box, it's an active community where customers propose new features, discuss improvements, and vote on ideas they want to see implemented.
This creates valuable data that guides Salesforce's development priorities. For example, when an idea receives 250 votes or 2,500 points, the development team evaluates it for implementation. This clear system shows customers that their input matters and helps Salesforce make choices based on real user needs. Learn more about data-driven decision making.
From Votes to Value: Prioritization and Implementation
Salesforce excels at turning customer votes into valuable features. Their voting system provides clear data on what customers want most, helping teams focus on high-impact improvements. This ensures resources go toward features that will make the biggest difference for users.
The company maintains open communication throughout development, keeping customers updated and gathering additional feedback. This ongoing conversation helps refine features and strengthens relationships with users.
Building a Feedback Loop for Continuous Improvement
The feedback loop created through the IdeaExchange does more than just guide individual features. It helps Salesforce spot broader trends and emerging customer needs, which shapes their long-term strategy. By consistently acting on customer input, they've built a culture focused on constant improvement.
This commitment to listening to customers has consistently improved their products and built stronger customer relationships. Tools like Derisky.ai can help other companies set up similar feedback systems to guide their product development.
Data Analytics: Advancing Modern Healthcare
Healthcare has made major strides in using data to improve patient care and treatment outcomes. Predictive analytics now helps hospitals and clinics make better decisions by analyzing past patient data to forecast future needs. For instance, analyzing admission patterns allows hospitals to identify patients at higher risk of readmission, enabling early intervention that prevents unnecessary hospital stays.
Optimizing Resource Allocation
Smart resource management is essential for hospitals to run efficiently while maintaining quality care. Data analytics helps healthcare providers make informed decisions about:
- Patient Volume Planning: Using historical data to forecast admission rates and staff needs
- Daily Operations: Analyzing peak times and supply requirements
- Supply Management: Tracking inventory to prevent shortages and reduce waste
Tools and Methods for Better Care
Healthcare organizations rely on several key technologies to blend clinical expertise with data insights:
- Electronic Health Records (EHRs): Give doctors quick access to complete patient histories
- Machine Learning Systems: Help identify health risks through pattern analysis
- Analytics Platforms: Combine clinical data with powerful analysis tools
Proper use of these tools requires careful attention to patient privacy regulations like HIPAA, ensuring data security while maximizing its benefits for care improvement.
Making a Real Difference
The impact of data analytics in healthcare is clear and measurable. Studies show that hospitals using predictive analytics have cut emergency visits by 20% and reduced costs by 15%. These results demonstrate how data-driven decisions lead to better patient outcomes and more efficient operations.
For healthcare organizations ready to improve through analytics, platforms like Derisky.ai offer practical guidance on implementing data strategies that enhance both patient care and operational performance.
Data-Driven Innovation in Financial Services: Beyond Basic Analytics
Financial institutions are fundamentally changing how they operate by embracing data-driven decision making. This approach goes far beyond reviewing historical performance – it enables organizations to spot emerging trends and develop smarter solutions for their customers. By applying advanced analytics across their operations, leading firms are reshaping everything from risk evaluation to personalized service delivery.
Modern Risk Assessment and Fraud Prevention
Risk management has evolved significantly through data-driven approaches. While traditional methods relied mainly on fixed rules and past data, today's systems use machine learning to analyze massive datasets and identify subtle fraud patterns. For instance, these tools can spot unusual transactions instantly and flag them for review, helping prevent major losses. By taking a proactive stance on risk, institutions can better protect both themselves and their clients.
Creating Personalized Customer Experiences
Financial firms now have unprecedented ability to understand and serve individual customers through data analysis. By studying specific financial behaviors and preferences, they can offer truly relevant services – from customized investment guidance to proactive support when it's needed most. This targeted approach helps build stronger relationships and keeps customers engaged for the long term.
Managing Security and Compliance
The expanded use of customer data requires careful handling of security and regulatory requirements. Financial institutions must protect sensitive information while following regulations like GDPR. They also need to ensure their algorithms remain transparent and unbiased. Success requires implementing strong data governance frameworks and maintaining high ethical standards.
Real-World Applications of Predictive Analysis
Financial organizations are putting predictive models to work in practical ways. These tools now help assess credit risk, forecast market movements, and optimize investment portfolios. For example, models can identify customers who might struggle with loan payments, allowing lenders to adjust terms or suggest alternatives early on. This leads to better outcomes for everyone involved.
Tools for Data-Driven Growth
Platforms like Derisky.ai provide financial institutions with practical tools to implement data-driven strategies. By combining AI capabilities with machine learning, these solutions help organizations develop effective data strategies and predictive models while maintaining security standards. This empowers firms to improve their operations and deliver better customer experiences based on solid data insights.
Building Your Data-Driven Decision Making Framework: A Practical Guide
Moving an organization to make decisions based on data brings clear business benefits, even though the transition process takes time and effort. Learning from successful companies that have made this shift can provide valuable insights. Let's explore the key elements needed to build an effective data-driven framework.
Creating a Data-Driven Culture
Building a data-focused culture starts with getting employees to see data as essential for decision-making. Research shows that companies using data to drive decisions are 23% more profitable than their competitors. Here's how to foster this culture:
- Educate and Train: Run regular workshops to help employees understand and work with data effectively
- Leadership Support: Have executives actively use and promote data-based approaches
- Share Success Stories: Highlight specific examples where data led to better business outcomes
Selecting the Appropriate Tools
The right data tools make a big difference in how well teams can access and analyze information. Focus on these key factors when choosing tools:
- Room for Growth: Pick solutions that can handle increasing data needs as you expand
- Easy Connections: Look for tools that work smoothly with your current systems
- Simple to Use: Choose options that all team members can understand and work with
Overcoming Common Obstacles
Most organizations face similar challenges when becoming more data-driven. Planning for these issues helps ensure success:
- Resistance to New Methods: Start with small pilot projects to show clear benefits and get buy-in
- Data Security: Set up strong protection policies while keeping data accessible to those who need it
Practical Framework Checklist
Follow these steps to put your data framework in place:
- Set Clear Goals: Define specific targets that connect data projects to business needs
- Gather Good Data: Identify and collect relevant, accurate information
- Analyze Well: Use appropriate tools to find meaningful patterns
- Act on Insights: Apply what you learn to make better decisions
- Keep Improving: Track results and adjust your approach based on what works
Implementation Timelines and Success Indicators
Track your progress with specific milestones and measurements. Look for improvements in:
- Decision quality: More accurate choices backed by data
- Cost savings: Reduced expenses from better-informed decisions
- Revenue growth: Increased sales from data-backed strategies
Building a data-driven organization takes time but brings lasting benefits like lower costs and higher profits. Derisky.ai offers tools to help organizations make better data-based decisions, manage risks effectively, and drive innovation. Learn how their solutions can strengthen your decision-making process today!