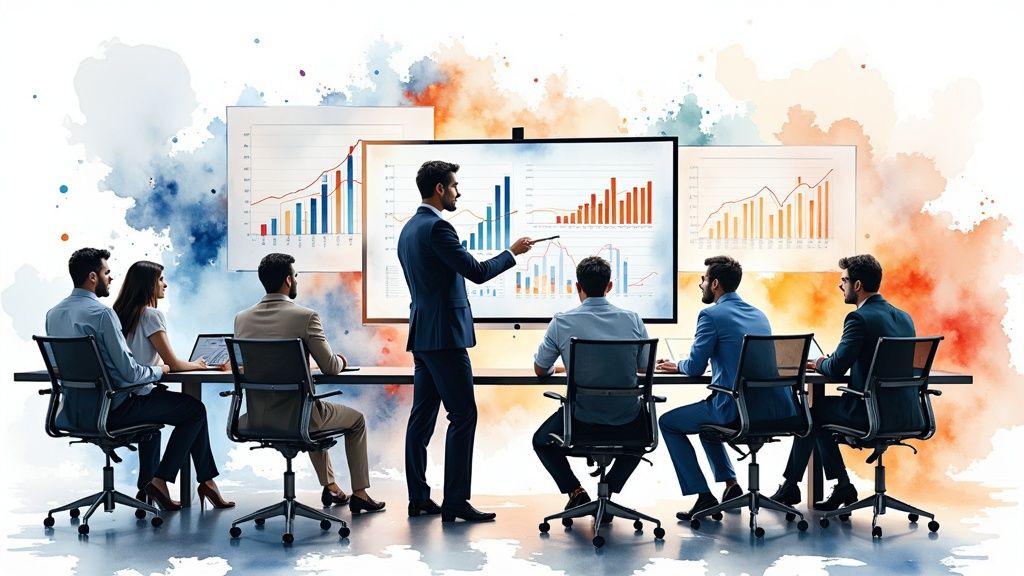
10 Powerful Data-Driven Decision Making Examples That Transform Business Results
The Revolution of Data-Driven Decision Making: Impact and Opportunities
Businesses are fundamentally changing how they make strategic choices. Rather than relying on instinct and guesswork, companies now base decisions on concrete data and analysis. This shift to data-driven decision making (DDDM) is reshaping how organizations plan, operate, and grow. But what's behind this change, and what possibilities does it create?
The sheer volume of available data has exploded in recent years. Digital systems now capture detailed information about customers, operations, and market conditions that was previously impossible to track. When analyzed properly, this wealth of data reveals valuable insights that can guide everything from product development to customer service. For example, companies can now analyze thousands of customer interactions to spot buying patterns and preferences, rather than making assumptions based on limited feedback.
Modern analytics tools have made it much easier to extract meaningful insights from data. Business intelligence platforms can now process massive datasets to identify trends and patterns that humans would miss. This helps companies predict outcomes more accurately, fine-tune their processes, and spot new growth opportunities. The result? Better-informed decisions about products, services, and target markets.
The business impact is clear and measurable. Research shows that companies embracing data-driven approaches are 5% more productive and 6% more profitable than their peers. Additionally, 77% of data and analytics professionals make DDDM a top priority to improve efficiency, reduce costs, and grow revenue. For more details, see these statistics about data-driven decision making. These numbers demonstrate how data analysis creates real business value across industries.
Seizing the Opportunities of Data-Driven Decision Making
Organizations that embrace DDDM can unlock several key advantages:
- Gain a Deeper Understanding of Customers: Analyze customer data to personalize offerings and improve service quality
- Optimize Operations: Identify process inefficiencies to reduce costs and boost productivity
- Develop Innovative Products and Services: Use data insights to guide new product development
- Make More Informed Strategic Decisions: Reduce risk by basing choices on solid evidence
However, success with DDDM requires more than just collecting data. Companies need proper tools, an analytical mindset throughout their culture, and team members who can effectively interpret data. When these elements come together, organizations can harness data's full potential to drive growth and gain competitive advantages. The businesses that master this approach will be best positioned to thrive and innovate.
Inside Google's People Analytics Revolution: Transforming HR Through Data
Google takes a unique approach to Human Resources by treating it as a science rather than an art. At the core of this strategy is their People Analytics Department – a specialized team that uses data to guide all people-related decisions. This marks a clear shift from traditional HR practices that relied mainly on gut feelings and conventional wisdom. By analyzing data systematically, Google uncovers valuable insights that help them make smarter choices about their workforce.
How Google Measures and Predicts Performance
The People Analytics team looks at many different aspects of employee and team performance. For example, they carefully study data from performance reviews and employee feedback to understand how managers impact their teams. This analysis reveals specific manager behaviors that lead to better results, helping Google focus their leadership development efforts where they matter most. The insights gained allow them to create targeted programs that improve performance across the organization.
Their data-driven approach has led to concrete improvements. When Google first analyzed their management practices, they discovered that teams with strong managers showed better performance, higher satisfaction, and longer employee retention. This finding prompted them to create the 'Great Managers Award' program and identify eight key behaviors that define excellent management. After implementing regular feedback surveys and updated training based on this research, manager approval ratings increased from 83% to 88%. Learn more about data-driven decision making here.
The Impact of Data on Google's HR Practices
Google's commitment to data has changed how they handle every aspect of talent management. From hiring new employees to developing leaders and keeping people engaged, each decision is backed by careful analysis. This approach helps them identify promising job candidates, spot future leaders, and create programs that actually work to improve employee satisfaction. Many other companies are now following Google's example, recognizing that data-based HR practices lead to better results. This shift has elevated HR from a support function to a strategic partner in achieving business goals.
Starbucks' Data-Driven Location Strategy: Mastering Market Expansion
When it comes to choosing store locations, Starbucks demonstrates how data-driven decision making can drive success in retail expansion. The coffee company carefully analyzes demographics, traffic patterns, and market conditions before opening new stores. This calculated approach helps them avoid costly mistakes while maintaining their competitive edge.
Integrating Data with Local Market Insight
The 2008 economic downturn forced Starbucks to close hundreds of stores, marking a shift in their location strategy. Instead of relying on gut feelings, they began partnering with analytics firms to examine hard data about demographics and traffic flow. But numbers alone don't tell the whole story. The company now combines this analysis with input from regional teams who understand local markets intimately. This balanced method helps them evaluate potential locations before making major investments. Find out more about their analytical approach here.
Tools and Methodologies Driving Expansion
Starbucks uses both predictive analytics and on-the-ground research to evaluate potential locations. Their key assessment criteria include:
- Population Density: They target areas with high concentrations of potential customers
- Traffic Flow: They measure daily foot and vehicle traffic past proposed sites
- Competitor Analysis: They study the distance to other coffee shops to avoid market saturation
The real strength of their strategy lies in how they merge concrete data with local expertise. This combination helps them not only choose the right locations but also tailor their stores to match local preferences and habits, increasing their chances of success in each new market they enter.
The Starbucks approach shows how combining careful analysis with human insight can help a company grow from a single coffee shop into a global brand. Their success offers valuable lessons for any business looking to expand strategically using data-driven methods.
Building Customer Experience Excellence Through Data Intelligence
Understanding and responding to customer needs has become essential for business success. Companies are finding that exceptional customer experiences drive growth and loyalty far more than traditional marketing. The key to delivering these experiences lies in using data intelligence – going beyond basic surveys to unlock deep insights about customer behavior and preferences.
Understanding the Customer Through Data
Modern businesses can now tap into rich streams of customer data from multiple touchpoints – website visits, purchase history, social media engagement, and more. When analyzed thoughtfully, this information reveals valuable patterns in how customers interact with a business. For instance, studying website navigation data helps identify where users get stuck or abandon their journey, allowing companies to streamline the experience and remove friction points.
Personalization at Scale with Predictive Analytics
The real power of data intelligence comes from enabling personalized experiences at scale. By applying predictive analytics to historical customer data, companies can anticipate individual needs and preferences. We see this in action when Netflix suggests shows based on viewing history or when Amazon recommends products that align with past purchases. This level of personalization helps build deeper connections with customers while driving business results.
AI-Powered Customer Interactions
AI technology is taking customer service to new levels of responsiveness and efficiency. Smart chatbots now handle common questions instantly, freeing up human agents to focus on complex issues that need a personal touch. AI tools also analyze customer feedback and reviews to spot trends and sentiment, helping companies improve their products and services. The combination of AI capabilities with human expertise creates a more complete customer support system.
Examples of Data-Driven CX Transformations
Here are some real-world examples of companies using data to enhance customer experience:
- Airlines: Using booking patterns and loyalty program data to create personalized travel offers and rewards
- Retailers: Combining in-store and online shopping data to optimize product placement and promotions
- Financial institutions: Analyzing transaction data to provide customized financial guidance and detect suspicious activity
These successes show how data intelligence helps build stronger customer relationships. With the right data tools and analytics capabilities, companies can better understand and serve their customers' needs. This customer-first approach, powered by smart use of data, is becoming essential for long-term business growth.
Next-Generation Supply Chain Management: Data-Driven Optimization
Supply chain complexity has reached new heights in recent years. Companies are responding by embracing data-driven decision making – moving beyond reactive approaches to proactively anticipate needs and build stronger operations.
The Power of Predictive Analytics
Smart predictive modeling is changing supply chain management fundamentals. By examining past data patterns, businesses can now forecast demand with greater accuracy and optimize inventory. Take the example of a retail store using historical sales data and weather forecasts to stock seasonal items – this helps maintain ideal inventory levels while reducing storage costs and stockouts. The result? Better sales and profits through smarter planning.
Real-Time Optimization with IoT Sensors
Internet of Things (IoT) technology has become essential for modern supply chains. Sensors placed throughout warehouses, vehicles, and products provide instant visibility into operations. This continuous flow of real-time data helps companies track shipments, monitor storage conditions, and manage logistics more precisely. For instance, managers can quickly spot bottlenecks, find better delivery routes, and ensure on-time customer deliveries.
Machine Learning for Enhanced Efficiency
The addition of machine learning takes data analysis to another level. These systems can spot hidden patterns in large datasets that humans might miss. This insight helps automate routine work, improve warehouse operations, and make demand forecasts more accurate. A key example is predictive maintenance – machine learning can spot potential equipment problems early, reducing costly downtime.
Examples of Data-Driven Supply Chain Management
Many companies are already seeing real benefits from data-driven supply chains:
- Retail giants: Using sales data to predict demand, set optimal prices, and suggest products to online shoppers
- Manufacturing companies: Following materials through production to maintain quality and use resources wisely
- Logistics providers: Using GPS and traffic data to plan better delivery routes and lower transport costs
These real-world cases show how data can significantly improve efficiency, reduce costs, and boost customer satisfaction. As companies continue to gather and analyze more supply chain data, they'll be better equipped to handle today's complex business challenges. The key is making smart use of available data to build supply chains that can adapt quickly to change.
Creating Your Data-Driven Decision Making Framework: A Practical Guide
Making smart business decisions requires a systematic approach grounded in data. This guide will help you build a framework to consistently make choices backed by evidence rather than just instinct. You'll learn practical steps for fostering a data-focused mindset, choosing effective tools, and building essential data skills across your organization.
Establishing a Data-First Culture
The foundation of good decision making starts with valuing data insights throughout your company. Rather than relying on hunches, encourage teams to find concrete evidence to support their recommendations. This shift begins with leadership modeling data-driven behaviors.
Set up regular meetings where teams share data-backed findings and discuss their business impact. When teams use data effectively, recognize and celebrate those wins. This positive reinforcement helps embed data-driven practices into your company's DNA.
Selecting the Right Analytical Tools
Success with data requires having the right tools for analysis. Your options range from basic spreadsheets to sophisticated analytics platforms. Choose tools based on your specific needs around data volume, technical capabilities, and business requirements.
Key factors to evaluate include user-friendliness, how well tools connect with your existing systems, and data visualization features. The goal is making data accessible to everyone who needs it for their work. When data tools integrate smoothly into daily tasks, teams are more likely to use them consistently.
Developing Data Literacy Across Teams
For data to drive decisions effectively, people need to understand how to interpret it. Invest in training that builds core data skills throughout your organization. While not everyone needs to be a data expert, all team members should grasp fundamental analysis concepts.
Focus on teaching practical skills like reading data visualizations, spotting meaningful patterns, and drawing reasonable conclusions. When everyone speaks the same data language, collaboration improves and decisions become more rigorous across all departments.
Implementing a Data-Driven Decision Making Process
Create a clear process for using data in decisions to ensure consistency. Your framework should outline specific steps for gathering, analyzing and applying data insights.
Here's a practical approach you can customize:
- Define the Problem: State exactly what business question you need to answer
- Gather Data: Collect relevant information from your key sources
- Analyze Data: Use your chosen tools to find patterns and insights
- Interpret Results: Draw clear conclusions from what the analysis shows
- Make Decisions: Choose actions based on the data evidence
- Monitor and Evaluate: Track results and adjust course as needed
This structured method helps teams stay objective and consistent in how they use data. It also creates a feedback loop to learn from past decisions and keep improving your approach.
Are you ready to transform your vague business ideas into secure investment opportunities, backed by the power of data? Derisky.ai can help bring data-driven clarity to your decision making process.