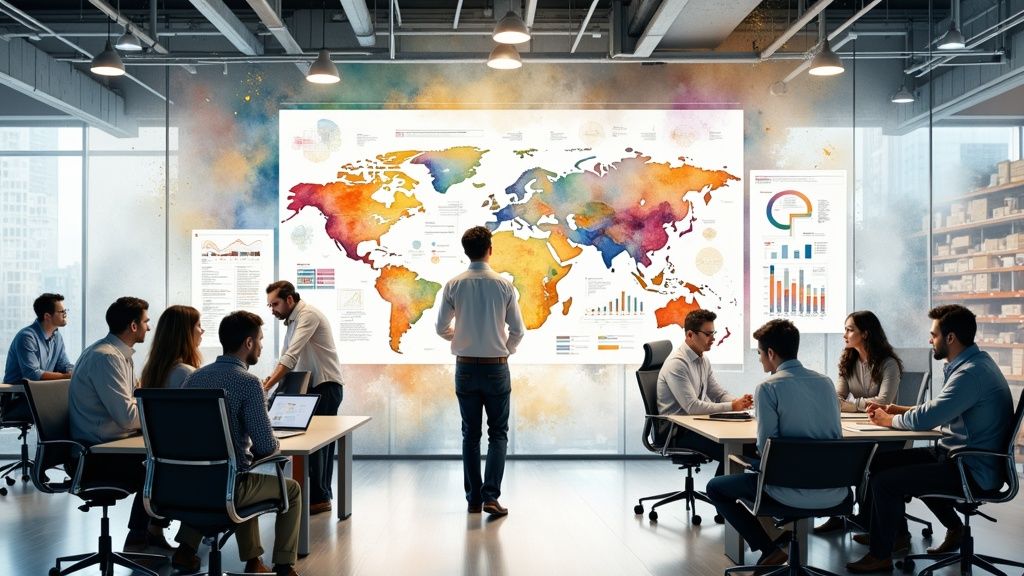
10 Powerful Data Driven Decision Making Examples That Transform Business Results
How Amazon Revolutionized Retail Through Data Intelligence
Data-driven decision making lies at the heart of Amazon's remarkable success story. By deeply understanding and predicting customer behavior, Amazon has fundamentally changed how retail operates. Their recommendation engine alone generates 35% of total revenue – a clear demonstration of how data insights directly impact business results. Let's explore the key ways Amazon uses data to drive growth and innovation.
The Power of Predictive Personalization
Amazon creates highly personalized experiences by analyzing multiple data points about each customer. Their algorithms look beyond just purchase history to consider browsing patterns, cart additions, time spent viewing items, demographics, and location. This comprehensive approach allows Amazon to present the most relevant products to each shopper at the right time. For instance, if you frequently browse cookbooks, you might see related kitchen gadgets or cooking ingredients recommended. This deep personalization helps build lasting customer relationships and drives sales growth.
Customer Segmentation: Understanding the Nuances of Needs
Amazon takes personalization further by grouping customers with similar characteristics and behaviors. This detailed segmentation allows them to deliver targeted marketing and product offerings that truly resonate. For example, price-sensitive shoppers might receive different promotions than premium brand buyers. Parents of young children see different product recommendations than college students. By understanding these distinct customer segments, Amazon can tailor its approach to match each group's specific interests and needs.
Optimizing Logistics and Operations
Behind the scenes, Amazon relies on data to perfect its operations and supply chain. Sales trends and demand forecasting help determine optimal inventory levels and warehouse locations. Real-time data guides decisions about shipping routes and facility layouts to maximize efficiency. This operational intelligence allows Amazon to minimize costs while still providing fast delivery and competitive prices. The company continuously analyzes performance metrics to identify bottlenecks and improve processes across its vast network.
A Continuous Cycle of Data-Driven Improvement
Amazon maintains its retail leadership by constantly refining its approach based on new data insights. The company actively collects customer feedback, studies behavior patterns, and tests new ideas to keep improving the shopping experience. This commitment to ongoing analysis and optimization ensures Amazon stays ahead as customer needs evolve. Rather than resting on past success, Amazon uses data to continuously enhance every aspect of its business – from product discovery to delivery.
Inside Walmart's Data-Driven Operations Revolution
Similar to Amazon's customer-first approach, Walmart has fundamentally changed its operations through data-driven decisions. By analyzing data across employee management, inventory control, store layouts, and product placement, the retail giant has maintained its strong market position while continuously improving customer experience.
Optimizing Employee Satisfaction and Performance
Understanding that satisfied employees create better shopping experiences, Walmart closely studied workforce data to measure how wages impact store performance. The analysis revealed clear connections between employee satisfaction and business results. This led Walmart to raise its minimum starting wage in 2016 – a decision firmly grounded in data rather than just good intentions. The change aimed to boost employee satisfaction, reduce turnover, and create better customer experiences.
Predictive Analytics in Inventory Management
Walmart's sophisticated inventory management system demonstrates how data analysis directly improves operations. Their approach combines multiple data streams to optimize stock levels and predict demand:
- Seasonal Demand Forecasting: By analyzing past sales patterns, Walmart stocks the right products at the right times, especially during peak shopping periods.
- Automated Reordering Systems: Real-time sales tracking triggers automatic reorders, which has cut stockouts by 40% and ensures products stay available.
- Weather Pattern Integration: The system factors in weather forecasts and local events to adjust store layouts. For example, stores prominently feature fans and summer items during heat waves.
Data Source | Impact on Operations |
---|---|
Historical Sales Data | Accurate seasonal demand forecasting |
Real-time Sales Data | Automated reordering, reduced stockouts |
Weather Patterns & Local Events | Optimized store layouts and product displays |
Driving Efficiency and Sales Performance
These data-informed strategies have produced measurable improvements. Better inventory management minimizes waste while ensuring product availability. Smart store layouts based on local data create more intuitive shopping experiences. As Walmart continues refining these approaches, they maintain their position as an industry leader by making decisions that benefit both the business and their customers.
Educational Innovation Through Predictive Analytics
Building on the success of Walmart's data strategy, Georgia State University (GSU) demonstrates another powerful example of using data to drive decisions and create meaningful change. GSU's story shows how analyzing student data can dramatically improve academic outcomes and graduation rates. Their proactive approach to supporting students through data analytics offers valuable lessons for other complex organizations looking to make better decisions.
Identifying At-Risk Students Early
GSU recognized that traditional advising methods often caught student issues too late. To address this, they developed a system that tracks multiple data points to spot struggling students before problems escalate. The system examines academic performance, class attendance, and even how students engage with online learning platforms. By connecting these different data sources, advisors gain a complete picture of each student's progress and challenges.
Creating a Proactive Support System
The system works like an early warning network for student success. When it detects potential issues, advisors receive immediate alerts so they can reach out and help. For example, if a student starts missing classes while their grades decline, an advisor can quickly step in to discuss any problems and connect them with relevant resources – whether that's tutoring, financial aid guidance, or counseling services. This shifts advising from reacting to problems to preventing them.
Targeted Interventions and Measurable Results
The impact of GSU's data-focused approach speaks for itself through an 8% increase in graduation rates. This improvement shows how using data to guide student support can create real results. By identifying which students need help and what kind of assistance works best, GSU ensures their resources go where they can make the biggest difference.
Implementing Data-Driven Decision Making in Education
Other schools can learn from GSU's framework for using data to help students succeed. Key steps include:
- Identifying Key Data Points: Select the most relevant information for predicting student success in your specific context, from academic records to engagement metrics
- Developing a Predictive Model: Build a system to analyze these data points and identify students who may need extra support
- Establishing Intervention Strategies: Create clear guidelines for how advisors should respond to alerts and provide appropriate help
- Continuous Monitoring and Refinement: Regularly check how well the system works and adjust based on results to maximize its impact
By following GSU's example, schools can transform their advising approach to better support students. Using data proactively helps identify and address challenges early, giving students the best chance to reach their full potential.
Healthcare's Data-Driven Transformation Story
The healthcare industry is making major strides in using data to improve patient care, following successful examples from retail and education sectors. The Cleveland Clinic offers an excellent case study of how analyzing patient information and treatment data can lead to better health outcomes. Their approach focuses on creating personalized care plans based on each patient's specific needs and circumstances.
Predictive Modeling for Improved Recovery
The Clinic uses predictive modeling to enhance post-surgery recovery results. By examining a patient's complete health profile – including medical history, existing conditions, and lifestyle factors – along with treatment data, medical teams can anticipate potential complications and create custom recovery plans. For instance, data analysis might show that patients with certain pre-existing conditions respond better to specific types of physical therapy after surgery. This allows doctors to address possible issues before they arise and design more effective recovery paths for each patient.
Reducing Readmissions Through Data Insights
The Clinic also uses data analysis to lower hospital readmission rates. By studying past readmission cases, they identify key patterns and risk factors, such as patient demographics, specific diagnoses, and post-discharge care practices. For example, their analysis revealed that patients with chronic conditions who lack reliable transportation often have higher readmission rates. This insight led them to implement targeted solutions like arranging rides to follow-up appointments or offering telehealth visits. These proactive steps help prevent unnecessary returns to the hospital.
Enhancing Emergency Response Systems
Data analysis also helps improve emergency department operations. The Clinic tracks patterns in ER visits related to time of day, day of week, and local events to predict busy periods. This information helps them schedule appropriate staffing levels and ensure adequate supplies are on hand during expected peak times. As a result, the emergency department can better handle sudden increases in patients while keeping wait times reasonable – critical factors in emergency care quality.
Optimized Resource Allocation and Capacity Planning
Looking at the bigger picture, the Clinic uses data to optimize overall hospital operations. They analyze patient flow patterns, typical length of stays, and treatment trends to forecast future bed needs and adjust staffing accordingly. This systematic approach has helped reduce average hospital stays by 15% while maintaining high care standards. Such improvements show how smart use of data can benefit both patients and hospital operations, creating a more efficient healthcare system that serves everyone better.
Measuring Success: The ROI of Data-Driven Decisions
To fully understand the impact of data-driven decision making, organizations must look beyond anecdotal evidence and focus on concrete, measurable results. By tracking specific metrics and analyzing quantifiable outcomes, companies can clearly see the value that data brings to their decision-making process.
Quantifying the Impact: Key Metrics for Success
While important metrics vary by industry, certain key performance indicators apply broadly across sectors. For instance, e-commerce companies often track conversion rates and average order values, while healthcare providers focus on patient outcomes and readmission rates. Here are the essential metrics that matter across industries:
- Revenue Growth: Organizations that effectively use data in decision making typically see revenue increases of up to 8%, making this a key indicator of success.
- Cost Reduction: Companies regularly achieve around 10% in cost savings through data analysis that identifies inefficiencies. Walmart's data-driven inventory management serves as a prime example.
- Improved Efficiency: The Cleveland Clinic demonstrated this by using data to reduce average hospital stays by 15% – showing how analytics can streamline operations.
- Customer Satisfaction: Amazon's recommendation engine, which drives 35% of their revenue, shows how data can create better customer experiences and boost loyalty.
- Employee Satisfaction: Walmart's success with data-informed wage adjustments proves that employee wellbeing directly impacts business results.
Tracking and Analyzing Metrics: Tools and Techniques
To effectively monitor these metrics, organizations need a structured approach and the right tools:
- Dashboards and Reporting Tools: Create a central view of key metrics to spot trends and track progress
- A/B Testing: Compare different approaches to determine which strategies work best
- Statistical Analysis: Move beyond basic data to uncover deeper patterns and cause-effect relationships
Demonstrating ROI: Building a Case for Data-Driven Decisions
To show the true value of data-driven decisions, start by establishing clear benchmarks before making changes. For example, if you want to improve customer support speed, first measure current response times. After implementing data-driven solutions, track the new metrics to measure improvement.
This approach works across all key metrics. By carefully documenting changes and improvements, you create solid evidence for the value of data-driven methods. When you can show concrete results – like faster response times or higher satisfaction scores – it becomes easier to justify investing in data tools and capabilities. This careful tracking turns insights into compelling evidence that data truly drives better business outcomes.
Building Your Data-Driven Decision Making Framework
Now that we've seen real examples of how data transforms decision making across industries, let's explore how to create an effective framework for your organization. This process involves more than just gathering data – it requires thoughtfully integrating data analysis into how your company makes choices at every level.
Identifying Valuable Data Sources
Start by pinpointing which data sources provide the most relevant insights for your specific goals. Consider internal sources like sales records, customer feedback, and website analytics, as well as external data from market research and industry reports. For example, an e-commerce company might focus on purchase history and browsing behavior, while a healthcare provider would prioritize patient records and treatment outcomes.
Establishing Meaningful Metrics
With your key data sources identified, define clear metrics that connect directly to your business objectives. Each metric should be measurable, trackable, and tied to specific outcomes you want to achieve. If your goal is better customer retention, you might track metrics like churn rate, customer lifetime value, and Net Promoter Score. This focused approach ensures your analysis efforts generate actionable insights.
Creating Effective Feedback Loops
Make data analysis an ongoing cycle rather than a one-time effort by establishing clear feedback loops. Regularly monitor your key metrics and assess how your decisions impact results. For instance, after launching a new marketing campaign based on data insights, track its performance against your goals. If results fall short, dig deeper into the data to understand why and adjust your approach accordingly.
Building a Data-Driven Culture
Success requires fostering a culture where data guides decisions at all levels. Help employees develop data literacy through training on analysis techniques and interpreting insights. When teams share a common understanding of data, it breaks down silos and promotes evidence-based collaboration. Encourage testing new ideas, measuring outcomes, and learning from both wins and setbacks. Companies that thrive with data are comfortable experimenting and adapting based on results.
Avoiding Common Pitfalls
Watch out for several challenges that can derail data initiatives. Poor data quality leads to faulty conclusions, so invest in proper data cleaning and validation. While historical data provides context, balance it with current market insights rather than over-relying on past patterns. Set clear timelines for analysis to avoid getting stuck in endless data review without taking action. Data should inform decisions, not delay them.
Ready to turn business concepts into proven opportunities and drive measurable results? Derisky.ai can help you achieve your goals with our powerful data-driven decision-making platform. Visit us today to learn how we can help you minimize risk, optimize your portfolio, and achieve breakthrough success.