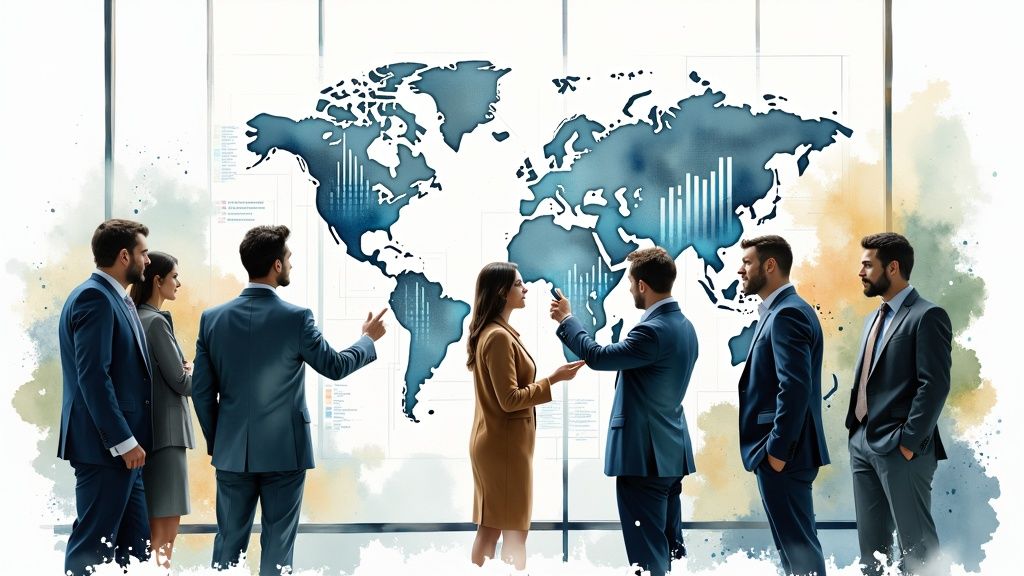
5 Powerful Data Driven Decision Making Examples: Transform Your Business Using Analytics
How Walmart Used Analytics to Transform Workforce Management
When Walmart faced serious workforce challenges like high turnover and low morale, they turned to data analysis rather than intuition to find solutions. By carefully examining employee and business data, they discovered key insights that would reshape their approach to workforce management.
The Link Between Happy Employees and Business Success
Through detailed analysis, Walmart uncovered clear connections between employee satisfaction and store performance. The data showed that higher employee satisfaction scores directly correlated with improved store cleanliness ratings and better customer feedback. For instance, stores with more satisfied employees consistently maintained higher cleanliness standards and received more positive customer reviews. This finding helped Walmart understand that investing in employee wellbeing wasn't just good for workers – it made strong business sense.
Making Smart Wage Decisions Based on Data
Armed with clear evidence linking wages to performance, Walmart began systematically increasing their starting pay rates. The numbers showed that while raising wages required upfront investment, it led to significant savings through reduced turnover costs and improved store operations. The results spoke for themselves – stores saw notable decreases in employee turnover alongside measurable improvements in cleanliness scores and customer satisfaction ratings. Sales figures also climbed as employee morale improved.
Measuring Success Through Key Metrics
Walmart developed a detailed system to track how employee-focused changes affected business outcomes. They monitored specific metrics like store cleanliness ratings, customer feedback scores, and employee satisfaction levels to understand the full impact of their initiatives. This careful measurement revealed impressive results – employee turnover dropped by 28%, while store performance metrics showed sustained improvement. The data proved that investing in workers through better wages and working conditions paid off through multiple business benefits.
This successful transformation shows how using data to guide workforce decisions can create positive change for both employees and the business. Walmart's experience offers valuable lessons for other organizations looking to improve their workforce management through careful analysis and evidence-based decision making.
Inside Google's Project Oxygen: Redefining Leadership Through Data
Google's quest to identify what makes a great leader wasn't based on gut feelings or popular management theories. Instead, they turned to data analysis through Project Oxygen to pinpoint specific behaviors that drive team success. Their goal was simple but ambitious: use data to identify and develop effective leadership practices.
Uncovering the Traits of Great Managers Through Data
The People Analytics team at Google took a systematic approach, analyzing thousands of performance reviews and employee feedback surveys. They looked for clear patterns connecting managerial behaviors to positive team outcomes. By examining both hard performance metrics and qualitative feedback, they identified key attributes that separated high-performing managers from others.
The Eight Habits of Highly Effective Google Managers
After extensive analysis, eight core behaviors emerged that consistently led to better team performance:
- Be a good coach: Providing regular feedback and guidance.
- Empower your team and don't micromanage: Trusting employees to own their work.
- Express interest in team members' success and personal well-being: Creating a supportive environment.
- Be productive and results-oriented: Focusing on achieving key objectives.
- Be a good communicator: Sharing information clearly and effectively.
- Help with career development: Supporting employee growth and advancement.
- Have a clear vision and strategy for the team: Providing direction and purpose.
- Have key technical skills: Possessing the expertise to guide the team effectively.
These behaviors weren't theoretical – they were proven through data to create positive outcomes within Google's specific culture and environment.
From Insights to Action: Transforming Leadership Development
Google didn't just document these findings – they put them into practice. The company developed focused training programs to help managers build these eight key behaviors. Each program provided practical tools and techniques grounded in real evidence about what worked, helping managers improve their effectiveness through concrete steps.
Measuring the Impact: Data-Driven Results
The results showed clear success. Manager approval ratings increased significantly after implementing these data-informed training programs. This proved that Project Oxygen wasn't just an academic study – it was a practical application that measurably improved leadership quality and team results. By studying what actually worked and building programs around those findings, Google created a model that other organizations could learn from to develop stronger leaders through careful analysis rather than assumptions.
Georgia State's Data Revolution in Higher Education
When faced with falling graduation rates, Georgia State University took an evidence-based approach rather than relying on assumptions. The university developed a sophisticated early warning system powered by data analytics to track student performance and provide timely support. This system has since become a model for how higher education institutions can use data to boost student success.
Building an Early Warning System
The university's monitoring system keeps tabs on over 40,000 students by looking at many different indicators beyond just grades. This comprehensive approach examines attendance patterns, completion of assignments, and even how often students use campus resources like the library. By considering these various data points together, Georgia State gets a much clearer picture of how each student is really doing in their studies.
AI-Powered Predictive Analytics
To make their system even more effective, Georgia State added artificial intelligence capabilities that can spot struggling students before traditional warning signs appear. For example, the system might notice when a student who regularly attends class starts having trouble with assignments, even if their grades are still okay. This allows the university to step in with help much earlier than they could before.
Targeted Interventions and Improved Outcomes
When the system identifies a student who needs support, it triggers specific assistance based on their situation. A student might be connected with a tutor, meet with an academic advisor, or get guidance about financial aid options. By matching the type of help to each student's needs, Georgia State has significantly improved its graduation rates. This shows how gathering and using data thoughtfully can lead to real improvements in student success.
Key Takeaways for Educational Institutions
Other schools can learn valuable lessons from Georgia State's experience. By using data analytics and predictive tools, colleges and universities can:
- Identify struggling students earlier: Catch problems before they become serious
- Provide personalized support: Give students the specific help they need
- Improve graduation rates: Help more students complete their degrees
- Optimize resource allocation: Focus resources where they'll do the most good
This case shows that data can guide meaningful improvements in education when used properly. By understanding patterns in student performance and taking action based on those insights, schools can help more students succeed. Georgia State's approach demonstrates that data analysis, when combined with targeted support, can make a real difference in educational outcomes.
Red Roof Inn's Weather Analytics Innovation Story
When most hotels see bad weather as a business challenge, Red Roof Inn saw an opportunity. Their creative approach to using weather data shows how companies can find new ways to grow by thinking differently about available information.
Identifying an Untapped Opportunity: Stranded Travelers
Red Roof Inn noticed a clear pattern – about 3% of flights get canceled each day, leaving thousands of travelers unexpectedly stranded at airports. Rather than compete for typical business travelers, they decided to focus specifically on helping these displaced passengers find a place to stay. This targeted approach gave them a unique position in the market.
Combining Data Sources for Precise Targeting
To make their strategy work, Red Roof Inn brought together two key data streams: flight cancellation records and weather forecasts. This combination helped them predict which airports would likely face disruptions due to weather events. For example, when a snowstorm hit Chicago, they could focus their efforts specifically on travelers stuck at O'Hare International Airport.
Real-Time Mobile Marketing: Reaching the Right People at the Right Time
The company then took action through targeted mobile ads. When bad weather hit, they sent promotional messages to phones in affected areas, offering nearby rooms at special rates. This timely outreach connected with travelers right when they needed help finding accommodation.
Measuring Success and Demonstrating ROI
The results showed their approach worked well. During periods of bad weather, Red Roof Inn saw double-digit increases in bookings at properties near affected airports. These clear numbers proved that their data-focused strategy delivered real business results. By identifying a specific problem, using data to find a solution, and measuring the impact, they created a successful model for data-driven decisions.
Lessons for Leveraging External Data
Other organizations can learn several key lessons from Red Roof Inn's success:
- Think creatively about data: Look for useful information sources that others might overlook
- Connect different data types: Combine various data streams to uncover new insights
- Act quickly: Build systems that can respond to changing situations
- Target specific customers: Focus marketing on clearly defined groups
- Track your results: Measure outcomes to show the value of data projects
This case shows how companies can gain an edge by finding smart ways to use publicly available information. Red Roof Inn's success came from looking beyond their own data to spot patterns that helped them serve customers better. Their story offers a practical example of how to make better business choices using data.
Uber's Real-Time Analytics Engine: A Deep Dive
Like Red Roof Inn's smart use of external data, Uber offers another compelling example of data-driven operations. The company's success depends on efficiently matching riders with drivers – a complex task requiring analysis of millions of real-time data points to keep their global network running smoothly.
Predicting Demand: Anticipating Rider Needs
A key part of Uber's strategy is forecasting where riders will need pickups. The system analyzes past ride patterns while factoring in real-world events like concerts, sports games, and weather conditions. For instance, when rain starts falling in a city, Uber's system detects the likely increase in ride requests and alerts nearby drivers to move to those areas. This proactive approach helps minimize passenger wait times while maximizing driver earnings through better positioning.
Dynamic Pricing: Balancing Supply and Demand
Uber's surge pricing model shows data-driven decision making in action. The system automatically adjusts fares based on real-time supply and demand in specific areas. When ride requests spike but driver availability is low, higher rates encourage more drivers to serve those busy zones. This automatic price adjustment helps maintain service even during peak periods by incentivizing drivers to meet increased demand.
Maintaining Optimal Service Levels: A Global Challenge
Providing consistent service across diverse markets poses unique challenges. Uber's analytics continuously track essential metrics like wait times, trip cancellations, and driver ratings across cities and time zones. This monitoring helps identify problem areas – for example, if a neighborhood shows high cancellation rates, deeper analysis might reveal issues like road construction or safety concerns that need addressing. By staying on top of these indicators, Uber can maintain service quality while adapting to each market's specific needs.
Technologies and Methodologies: The Engine Behind the Scenes
Uber's real-time capabilities run on robust technical foundations. The company uses Hadoop and Apache Spark to process massive datasets efficiently. Machine learning models help predict demand patterns and optimize driver dispatching. The system also employs advanced location analysis to understand movement flows and identify high-demand zones. This combination of technologies and careful data analysis creates a responsive platform that adapts to changing conditions. For other organizations looking to build similar capabilities, Uber's approach shows how combining solid technology with smart data strategy can significantly improve operations and customer satisfaction.
Building Your Data-Driven Decision Making Framework
The successes of companies like Walmart, Google, Georgia State, Red Roof Inn, and Uber show how data can drive smart business decisions across many different industries. But putting these ideas into practice requires a clear plan and structure. Here's a practical guide for creating a data-driven approach that helps your organization make better decisions based on real insights.
Identifying Analytics Opportunities
Start by pinpointing specific areas where data analysis can solve real business problems. What are your key challenges and goals? Maybe you want to keep more customers, spend marketing dollars more effectively, or make operations run more smoothly. Look at which business processes generate useful data and where new data collection could provide answers. Focus on opportunities where data insights can directly impact your most important objectives.
Selecting Meaningful Metrics
After identifying opportunities, choose metrics that directly connect to your goals and can be measured accurately. For example, if you aim to boost sales, track conversion rates, average order value, and how much customers spend over time. Be selective – monitoring too many metrics creates confusion. Pick a focused set of key performance indicators (KPIs) that matter most for your specific objectives.
Building Robust Data Capabilities
Effective data-driven decisions require the right foundation of tools and skills:
- Data Storage and Management: Put systems in place to collect, organize and protect data efficiently
- Analytics Tools: Get the right software to analyze data patterns and create clear reports
- Data Literacy: Train employees across the organization to work with and understand data, not just specialists
Creating a Data-Driven Culture
Building data into your company culture goes beyond just tools and technology. Make data transparency a priority, base decisions on evidence, and recognize teams that use data well. Share insights regularly between departments to spark new ideas. Provide training so everyone feels confident working with data to make informed choices.
Overcoming Common Challenges
Most organizations face some obstacles when becoming more data-driven. Here's how to handle common issues:
- Data Silos: When data gets stuck in different departments, it's harder to see the full picture. Create central systems that make data sharing easier.
- Data Quality: Bad data leads to bad decisions. Set clear standards and processes to keep data accurate and complete.
- Resistance to Change: Some people may hesitate to adopt new data-driven methods. Address concerns openly and provide support through the transition.
This framework gives you the building blocks for data-driven decision making. By methodically finding opportunities, choosing the right metrics, and developing your data capabilities, you'll help your organization make smarter choices backed by real evidence.
Looking to turn business ideas into measurable opportunities? Derisky.ai helps companies track innovation performance, test ideas systematically, and make smarter investment choices using data. Learn more at https://derisky.ai.